Home
EPBABI Course Curriculum
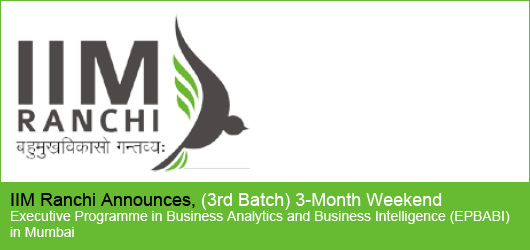
Course Curriculum for Executive Programme in Business Analytics & Business
Intelligence (EPBABI)
Modules
Module - I Module-II Module-III
Statistics for Business
Analytics
Random Analytic Processes Time-Series Analysis
Module-IV Module-V Module - VI
Data Mining and
Business Analytics
Analytics for Business Process
Improvement
Data Warehousing and
Business Intelligence
Module-VII Module-VIII Module-IX Module-X
Optimization
Analytics
Business Analytics in
Global Operations
Predictive Analytics Business Analytics
Applications
Module XI
Hands on experience
Course Contents for
Executive Programme in Business Analytics & Business
Intelligence (EPBABI)
Module1: Statistics for Business Analytics
Descriptive Statistics
Introduction: Nature of Statistics, Uses of Statistics, Statistics in relation to other disciplines,Types of Data: Concepts of population and sample, quantitative and qualitative data, cross-sectional and time-series data, discrete and continuous data, different types of scales. Presentation of data: Construction of tables with one or more factors of classification, diagrammatic representations, frequency distributions and cumulative frequency distributions, relative frequency distributions Univariate data – different measures of location, dispersion, relative dispersion: mean absolute deviation (MAD), range, quartile deviation, interquartile range, standard deviation, basic concepts of moments, ; central and noncentral moments; skewness and kurtosis, Lorenz curve
Probability and Sampling
Concepts of probability-classical and empirical approaches; theorems of probability; stqtistical independence of events; Central Limit Theorem;axiomatic approach to probability; random variables; conditional probabilities-Bayes’ Theorem; probabaility mass functions and probability density Functions ;probabailty distributions-Binomial,Poisson and Normal Distributionsother distributions such as Student’s t-distribution and Snecedor’s F-distribution.
Hypothesis Testing and Statistical Inference:
Introduction to hypothesis testing; Concepts of null and alternate hypothesesconcept of test of significance; confidence intervals - critical value method and p-value method ; point and interval estimates; maximum likelihood estimation (MLE);tests for goodness of fit; Type I and Type II errors; power of a test; applications of hypothesis testing in business decision-making
Non-parametric methods:
Introduction; non-parametric estimation of location and dispersion; tolerance intervals; non-parametric tests for location: one-sample sign, one-sample Wilcoxon signed-rank, paired-sample sign, paired-sample Wilcoxon signed-rank tests
Multivariate Analysis & Techniques:
Characteristics of multi-variate data, elementary ideas of multi-variate distributions;; introduction to multiple regression and multiple correlation; some results relating to multiple regression and multiple correlation; partial correlation; relations linking partial regression and partial correlation
Module 2: Random Analytical Processes
Random variables:
probability spaces, probability measures etc. Random variables, conditional expectation, characteristic functions, limits theorems.
Stochastic processes
Basic definitions.Brownian motion.Stationary processes. Other examples of stationary processes; Brownian motion in the stock market
Markov processes
Basic definitions.The Chapman-Kolmogorov equation.The generator of Markov process and itsadjoint.Ergodic and stationary Markov processes.
Stochastic Differential Equations
Basic properties of SDEs.Itô’sLemma; Numerical solution of SDEs.
Monte-Carlo Simulation & Sensitivity Analysis
Module3: Time Series Analysis
Introduction to time series; organizing data for analysis
Examples, simple descriptive techniques, trend, seasonality, the correlogram.
Probability models for time series
Moving average (MA), Autoregression (AR), ARMA(Autoregressive Moving Average) and ARIMA (Autoregressive Integrated Moving Average) models.Estimating the autocorrelation function and fitting ARIMA models.
Forecasting:
Exponential smoothing.Forecasting from ARIMA models.
Stationary processes in the frequency domain:
The spectral density function, the periodogram, spectral analysis
State-space models:
Dynamic linear models and the Kalman filter technique
Module 4: Data Mining & Business Analytics
Introduction to Business Anaytics and Data Mining: What is data mining? KDD vs
Data Mining (DM), DM Tasks, DM and analytics Application Areas
Association Rule (AR): Market Basket Analysis, Representation of an AR, Strength of
AR, Support, Confidence, and Lift, Generalized Association Rule (numeric, categoric, temporal, spatial etc.), Case study on use of association rules in Market Basket Analysis and Inventory Management
Clustering and Classification: Difference between clustering & classification, Discussion on clustering techniques, Case discussion on the application of clustering, Classification using Decision Tree, Classification using Artificial Neural Network (ANN), case discussion
Feature Selection: Feature selection for Data Reduction, Application of feature selection
in classification
Sequence Mining: Sequence rules, Applications of sequence rules
Text Mining and Web Mining: Importance of text and web mining, various types of web
mining, Applications of text and web mining
Module 5: Analytics for Business Process Improvement
- Business Processes, Process Flow Diagram
- Performance and capability of a process, Process Capability Indices,
- Lean Concepts, Six Sigma, DMAIC, Lean Six Sigma
- Identification of defects in various business processes
- Random vs. Assignable Causes: Use of probability in detecting assignable causes
- Testing for Statistically Significant Change in the Process using variables data: (i) sample vs. population, (ii) between two samples
- Testing for Statistically Significant Change in the Process using proportional data: (i) sample vs. population, (ii) between two samples
- Testing for Statistically Significant difference in two or more number of processes/treatments using ANOVA and ANOM, One -Way ANOVA,
Factorial ANOVA.
Module 6: Data Warehousing & Business Intelligence
Introduction to descriptive analysis:
Data Warehousing: What is a data warehouse? Elements of a Data Warehouse Data Warehouse (DW) vs. Database, Commercial Importance of data warehouse, Fundamentals of Multidimensional data model, Fact/measure, what is dimension? DW Architecture, Data Marts, Virtual Data Warehouse, Metadata, Multidimensional Representation of data: Dimension Modeling & Hierarchy, Lattice of Cuboids, Summary
Measures
OLAP operations:
Slicing & Dicing, Drill-up & Drill-down, Drill within & Drill Across, Pivot
Warehouse Schema:
Normalization vs Dimensional Modeling, Star Schema, Snowflake Schema and Fact Constellation
Data Warehouse Implementation:
Efficient Computation of Data Cubes, Indexing OLAP Data, Backend Processes of ETL
DW issues in Retail sale:
Promotion Dimension, Degenerate Dimension, Retail Schema Extensibility DW issues in Inventory Management: Inventory Periodic snapshot, Inventory Transactions, Inventory Accumulating Snapshot
DW issues in Procurement:
How to handle Slowly Changing Dimensions (SCD)
DW issues in CRM:
How to handle Large Changing Dimensions (LCD), Discussion on DW in Banking,
Insurance, Healthcare, Education etc.
Module7: Optimization Analytics:
Introduction to Operations Research (OR):
Linear Programming Problem (LPP):
Formulating a Linear Programming Problem; Graphical and Algorithmic Approaches; feasible solutions; concept of Basic Feasible Solution (BFS); duality theorems; Simplex Algorithm for solving LPPs; application of LPP in decision-problems; sensitivity analysis;
Transportation Problem:
Formulating Linear Programming to problems in transportation Applications of Linear Programming to problems in transportation; Solution algorithms such as North-West Corner Rule and Vogel’s Approximation Method (VAM);,MODI.
Assignment Problem:
Defining Assignment Problem in OR; Solution Technique for Assignment Problem; Assignment scheduling and task planning; basic ideas of network models
Introduction to Non-Linear Programming and Integer Programming:
Discussion on the applications
Queuing Theory: Discussion on the applications
Module8: Business Analytics in Global Operation
Drivers of Global Operations
This section describes the various drivers of global operations for an enterprise.
Growth vs. Stagnation
Stagnation in the Enterprise’s home turf results in looking for greener pastures elsewhere for growth. This is one of the key drivers for going global.
New Opportunity
New Opportunities, either new products/services or new partnerships or just mere extension of current product portfolio can also be a driver for going global.
Cross-Border M&A
New partnerships or diversification through mergers & acquisitions is yet another driver for going global.
SWOT Analysis vs. PESTAL Analysis
Deciding to go global is definitely not an easy one. Lot of preparations has to be made before diversifying in international territory It has to begin with an introspection followed by an extra-spection which needs to complement each other. Examples of confidential information include but are not limited to: company private, corporate strategies, competitor sensitive, trade secrets, specifications, customer lists, and research data. Employees should take all necessary steps to prevent unauthorized access to this information.
SWOT Analysis
This is an introspection that an organization needs to do to decide what are its Strengths and Weaknesses and what are the Threats and Opportunities in the market place.
PESTEL Analysis
The Political, Economic, Social, Technological, Environmental, and Legislative environment prevalent in various countries is going to impact on any business decisions. Hence it is a necessity to analyze all these before deciding on International engagements.
Information Pyramid for Global Operations Decision Making
The decision to go global is obviously the CXO’s prerogative. Such decisions are mostly going to be strategic in nature and some will also be tactical in nature as well.
Strategic Decisions
The data points needed to make strategic decisions are by nature going to be rather fuzzy and it will be dependent on unstructured data, most of which will probably come from sources external to the organization.
Tactical Decisions
Whereas, the data points needed to make tactical decisions are by nature going to be more defined and it will be dependent on structured data majority of that will be sourced internally while some of them will still come from external sources.
Key Result Areas (KRA) for Global Operations
Country Strategy
Deciding which all country to have footprint and then to have country rollout plan is a crucial element in the success of a global operation.
Consumer Behavior Analysis
Understanding the consumer behavior across geography will also be a key to successful business. Whether it is B-2-B or B-2-C, knowing the end customer is very vital.
Supply Chain Analysis
Understanding the Supply Chain Ecosystem is the third important area that has potential impact on any decision making related to global operations.
Competitor Analysis
Understanding the Competition is the fourth important area that has to be analyzed in great details before jumping on the Global operations band wagon.
International Human Capital Strategy
Whether an organization decided to send their own talents to new soil or chose to use the cross-border M&A route to go global, International Human Capital management is going to be a key aspect when it comes to overall integration and operation.
Extraneous Factors that affect Global Operations
Regulatory & Statutory Requirements
Be aware about various regulatory and statutory requirements each new Country may have is very important.
Generally Accepted Accounting Principles
Be aware about the accounting standards, payroll regulations that may be prevalent in each new Country is also very important.
Localization Requirements
Whether using “Local Sourcing & Global Reach” or the reverse model i.e. “Global Sourcing & Local Reach” understanding the nuances of local needs and adopting to such diversity is going to be a key for the success of all global initiatives and operations.
Data Collection Methods for Global Operations Analysis
Market Research
Using a Market Research agency having global operations may be a key. This needs to be complemented with Local Market Research data as well.
Social Media
Using a Social media as a potent medium of collecting data may be very useful.
Module 9: Predictive Analytics
- Forecasting with ( i) Simple Linear Regression Model, (ii) Multiple Linear Regression Model
- Logistic Regression: with application
- Conjoint Analysis: with application
- ANOVA and MANOVA: with application
Module 10: Business Analytics Applications
Business analytics applications in:
- Marketing and CRM
- Operations and Supply Chain
- Banking & Finance
- HR
- Miscellaneous Services like Retailing, Hospital, Education etc.
Module 11: Hands-on-experience
- Predictive Analytics
- Data Cuboids and Business Intelligence (BI)
- Data Mining
Contact
Office of Admission Processing
Aegis School of Business and Telecommunication
Cell +91 9819008153/ 9987299358
Phone: +91 (0)22 32185553|
Email: [email protected]
For more information: www.aegis.edu.in
Address
Mahesh, Plot No.37, Sector 15, | Central Business District (CBD), Belapur | New Mumbai | MH | 400614 | India |